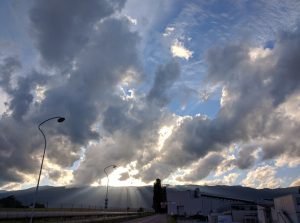
I just spent two weeks at CERN. Due to limited travel funds this year, I had to keep my summer time here limited to save money for other trips and my research leave in the spring of 2017. Nonetheless, this was an incredibly productive pair of weeks. I’ll reflect a little on what was accomplished and look ahead at the promise of much good science to come this year from the Large Hadron Collider (LHC).
Being at CERN in the summer is extremely important to me. First, it’s the beating heart of my field – collider-based particle physics – these days. It is home to many such operating experiments, many of which utilize the incredible LHC. The second reason that this is so important to me is that half the SMU colleagues I work with are physically located at CERN, so I spend most of my time interacting with them purely via email, Skype, or Vidyo. Face-time (actual face-time, not that silly Apple app) is crucial to progress in science. To me, at least, as convenient as internet-based collaborative tools are, there is still no substitute for being in the same office, interrupting each other with questions, and even making time for comaraderie.
Our ATLAS group’s previous grant cycle ended in March, and our new grant started on April 1. While I think that I reviewed well in the comparative panel reviews that assess grant proposals, it seems that it was typical for most US institutions – especially the Universities – to see some reduction in LHC-related funding as the US funding agencies and our domestic program pivot toward ongoing and new domestic programs in neutrino physics, dark matter searches, and astrophysics and astronomy. This has essentially led to a reduction in, among other things, travel funds. Instead of spending 4-5 weeks at CERN, I cut my time in half so I can make another short trip in the fall when some of our people will relocate to CERN.
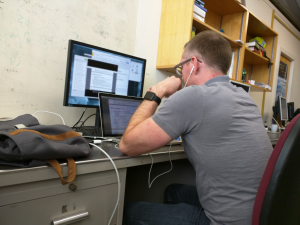
The two weeks were quite focused. My primary physics interest is in the search for the extremely important Higgs boson decay . My senior graduate student is involved in this analysis and is, as one would expect, the much more active of the two of us in day-to-day analysis activities. Being at CERN allowed me to provide mentoring and learn more about his typical daily activities and distractions, as well as to interact with leadership in this analysis effort. Of course, all physics analyses are feeling tremendous pressure to make summer conference deadlines and ours is not immune from that. Personally, I believe that measuring this HIggs decay (a feat which neither CMS nor ATLAS achieved directly in Run 1) is one of the most imporant measurements that will come from Run 2, since it will likely be the first direct observation (should this decay actually exist) of a Higgs-quark interaction. Higgs-lepton interactions had clearer evidence in Run 1 presented by both ATLAS and CMS via the process
.
Related to this primary physics interest are our core technical and physics support activity areas, bottom-quark-initiated jet triggers and flavor-tagging (the process of discerning what quark initiated a jet via experimental observational methods). Here, I have involved not only a senior graduate student but also a post-doctoral researcher. This work is never over, because a running experiment and active physics analysis program always demands improvement, upgrade, and repair. “Triggers” are the algorithms that make decisions, in real-time, about which proton-proton collisions to keep and which to reject. This is a crucial system; it’s the last line of defense before data are written to disk for use by physicists, and if it’s overly harsh it can throw out interesting events . . . and we might never even know. You can imagine that getting this right is important to the collaboration. “b-jet triggers,” which we work on, are part of a large suite of such trigger algorithms that are managed by hundreds of physicists on ATLAS. Our current work in b-jet triggers is focused on tuning the algorithm to make the best use of information available at the level of computing where the algorithm runs.
In flavor-tagging, my senior graduate student has been involved in efforts to reduce the number of “nuisance parameters” associated with the corrections that make simulated bottom-quark jets look more like the actual jets in data. This is difficult statistical business. You can, of course, choose to use a reduced set of such nuisance parameters . . . ones you believe contain “most” of the relevant information. But in doing this you can throw away crucial correlations between nuisance parameters, and in doing so (and in doing the reduction in the first place) you can misrepresent the actual total error in your own physics analysis. So we’ve been trying to find ways to do this reduction while preserving sufficient information. Reducing the number of nuisance parameters improves the stability of data-interpretation efforts, but may lose important uncertainty in the process and then misrepresent our knowledge of the data. Getting this right is important to me and my student.
While the above activities were the bulk of the important things – the things that really make or break this summer – I was also involved in a very engaging side-project with another SMU faculty member and a post-doc, as well as a small group of SMU graduates students and an undergraduate. We’ve been trying to learn about the exact features of very rare Higgs production mechanisms and then design, with a ground-up or top-down approach, a way of identifying those Higgs Bosons while rejecting the copious non-Higgs processes (“background”) produced by the LHC. This has been immense fun. We’ve been meeting a few times per week showing reports on progress, swapping code and ideas, and now we are beginning to document what we’ve learned. I took a side-step, encouraged by my senior graduate student, into advanced statistical classification using excellent tools like Pandas, Seaborn, and SciKit-Learn [1]. All of these are available thanks to the Python programming language and a very active community of computer scientists, statisticians, and scientists from many disciplines – not just physics. There are tools available in standard high-energy physics software, but I have found them a bit bloated and clunky . . . mostly because of how they are implemented in C++. I have preferred Python for years, and used it to great effect in Run 1 for my own physics analysis. Now I am trying to teach myself some new tricks, and it has been extremely rewarding.
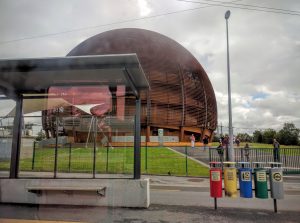
So the past two weeks have been hyper-productive. There was progress on physics and technical efforts. There was progress on a fun summer side-project that may bear fruit in the fall. I got a lot of face-time with people at CERN, and not just the members of my own group. I even did a LOT of running to enjoy the outdoors (even while it was raining) and manage stresses and pressures common to life on a large collaboration. I had a great time.
Now I am on a train to Paris. My flight to Dallas is tomorrow morning. I am looking forward to being home, but I am pleased to be able to known that my “work in the field” at CERN has been as productive as I could have wanted . . . if not more.
[1] Pandas: http://pandas.pydata.org/, Seaborn: http://stanford.edu/~mwaskom/software/seaborn/index.html, SciKit-Learn: http://scikit-learn.org/stable/index.html